Free Downloads
Bayesian Signal Processing: Classical, Modern And Particle Filtering Methods (Adaptive And Cognitive Dynamic Systems: Signal Processing, Learning, Communications And Control)

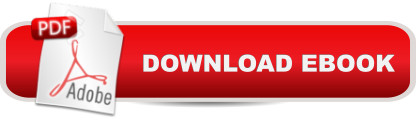

New Bayesian approach helps you solve tough problems in signal processing with ease Signal processing is based on this fundamental concept—the extraction of critical information from noisy, uncertain data. Most techniques rely on underlying Gaussian assumptions for a solution, but what happens when these assumptions are erroneous? Bayesian techniques circumvent this limitation by offering a completely different approach that can easily incorporate non-Gaussian and nonlinear processes along with all of the usual methods currently available. This text enables readers to fully exploit the many advantages of the "Bayesian approach" to model-based signal processing. It clearly demonstrates the features of this powerful approach compared to the pure statistical methods found in other texts. Readers will discover how easily and effectively the Bayesian approach, coupled with the hierarchy of physics-based models developed throughout, can be applied to signal processing problems that previously seemed unsolvable. Bayesian Signal Processing features the latest generation of processors (particle filters) that have been enabled by the advent of high-speed/high-throughput computers. The Bayesian approach is uniformly developed in this book's algorithms, examples, applications, and case studies. Throughout this book, the emphasis is on nonlinear/non-Gaussian problems; however, some classical techniques (e.g. Kalman filters, unscented Kalman filters, Gaussian sums, grid-based filters, et al) are included to enable readers familiar with those methods to draw parallels between the two approaches. Special features include: Unified Bayesian treatment starting from the basics (Bayes's rule) to the more advanced (Monte Carlo sampling), evolving to the next-generation techniques (sequential Monte Carlo sampling) Incorporates "classical" Kalman filtering for linear, linearized, and nonlinear systems; "modern" unscented Kalman filters; and the "next-generation" Bayesian particle filters Examples illustrate how theory can be applied directly to a variety of processing problems Case studies demonstrate how the Bayesian approach solves real-world problems in practice MATLAB notes at the end of each chapter help readers solve complex problems using readily available software commands and point out software packages available Problem sets test readers' knowledge and help them put their new skills into practice The basic Bayesian approach is emphasized throughout this text in order to enable the processor to rethink the approach to formulating and solving signal processing problems from the Bayesian perspective. This text brings readers from the classical methods of model-based signal processing to the next generation of processors that will clearly dominate the future of signal processing for years to come. With its many illustrations demonstrating the applicability of the Bayesian approach to real-world problems in signal processing, this text is essential for all students, scientists, and engineers who investigate and apply signal processing to their everyday problems.

File Size: 11609 KB
Print Length: 472 pages
Publisher: Wiley-IEEE Press; 1 edition (September 21, 2011)
Publication Date: September 21, 2011
Sold by: Digital Services LLC
Language: English
ASIN: B005PS50XY
Text-to-Speech: Enabled
X-Ray: Not Enabled
Word Wise: Not Enabled
Lending: Not Enabled
Enhanced Typesetting: Enabled
Best Sellers Rank: #1,206,960 Paid in Kindle Store (See Top 100 Paid in Kindle Store) #83 in Kindle Store > Kindle eBooks > Nonfiction > Science > Physics > Waves & Wave Mechanics #151 in Books > Computers & Technology > Hardware & DIY > Microprocessors & System Design > Control Systems #446 in Books > Science & Math > Physics > Waves & Wave Mechanics

Bayesian Signal Processing: Classical, Modern and Particle Filtering Methods (Adaptive and Cognitive Dynamic Systems: Signal Processing, Learning, Communications and Control) Digital Signal Processing: with Selected Topics: Adaptive Systems, Time-Frequency Analysis, Sparse Signal Processing Learn: Cognitive Psychology - How to Learn, Any Skill or Subject in 21 Days! (Learn, Learning Disability, Learning Games, Learning Techniques, Learning ... Learning, Cognitive Science, Study) Bayesian Methods for Hackers: Probabilistic Programming and Bayesian Inference (Addison-Wesley Data & Analytics) Adaptive Filtering Prediction and Control (Dover Books on Electrical Engineering) Modeling and Control of Discrete-event Dynamic Systems: with Petri Nets and Other Tools (Advanced Textbooks in Control and Signal Processing) Ending Spam: Bayesian Content Filtering and the Art of Statistical Language Classification Learning: 25 Learning Techniques for Accelerated Learning - Learn Faster by 300%! (Learning, Memory Techniques, Accelerated Learning, Memory, E Learning, ... Learning Techniques, Exam Preparation) Biosignal and Medical Image Processing (Signal Processing and Communications) Digital Signal Processing in Communications Systems Dynamic Programming and Optimal Control, Vol. II, 4th Edition: Approximate Dynamic Programming Signal Processing Algorithms in Fortran and C (Prentice-Hall Signal Processing Series) Multidimensional Digital Signal Processing (Prentice-Hall Signal Processing Series) Digital Signal Processing with Examples in MATLAB®, Second Edition (Electrical Engineering & Applied Signal Processing Series) Discrete-Time Signal Processing (3rd Edition) (Prentice-Hall Signal Processing Series) Hack Your Mind to Become Bulletproof: How to control perceptive filtering, create happiness on command, interrupt destructive patterns, unlock the power ... to Create a New Future Series Book 1) Deep Learning: Methods and Applications (Foundations and Trends(r) in Signal Processing) Power Electronic Converters Modeling and Control: with Case Studies (Advanced Textbooks in Control and Signal Processing) Dynamic Offset Compensated CMOS Amplifiers (Analog Circuits and Signal Processing) Machine Learning: A Bayesian and Optimization Perspective (Net Developers)
